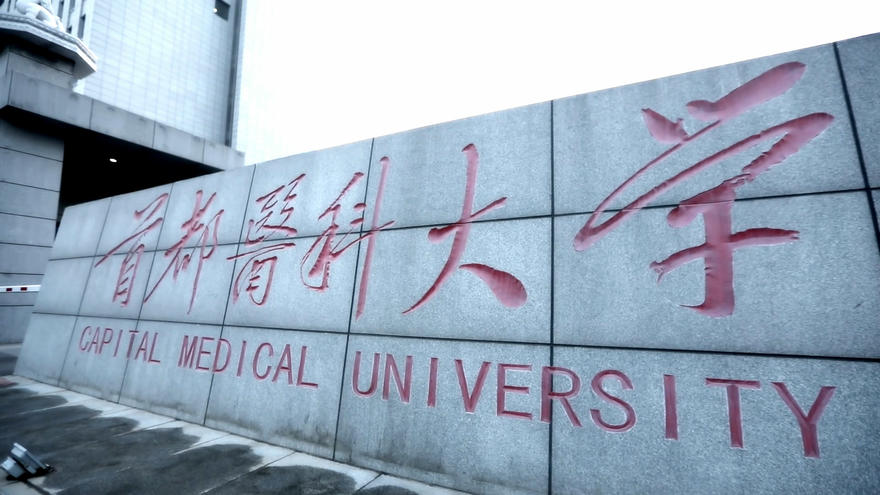
About This Course
To present a careful treatment of the principal topics of linear algebra and to illustrate the power of the subject through a variety of applications. Topics including: systems of linear equations, determinants, matrices, vector spaces, linear transformations, eigenvalues and eigenvectors, and quadratic forms. Linear algebra is suitable for all students majoring in science or mathematics, or any other courses of study requiring it.
After completing this course, students will be able to understand the basic methods and techniques in linear algebra. This course also helps students develop their abilities in abstract thinking and logical reasoning.
Linear Algebra serves as a prerequisite for many courses, including numerical analysis, ordinary differential equations, partial differential equations, regression analysis, financial mathematics and financial engineering and etc.
Requirements
The student should have some familiarity with the basics of differential and integral calculus.
Course Staff
Professor, School of Mathematics and Statistics, Beijing Jiaotong University, Beijing, China
Major:
Optimization theory, algorithms and applications
Educational background:
1997-2001, Normal School, Qingdao University, Bachelor Degree
2001-2004, Beijing Jiaotong University, Master Degree
2004-2008, Hirosaki University, Japan, PhD Degree
2018-2019, University of California, Davis, USA, Visiting Scholar
Representative papers:
[1] X. Chen, C. Zhang, and M. Fukushima, Robust solution of monotone stochastic linear complementarity problems, Math. Program. 117 (2009), 51-80.
[2] C. Zhang and X. Chen, Smoothing projected gradient method and its application to stochastic linear complementarity problems, SIAM J. Optim. 20 (2009), 627-649.
[3] C. Zhang, X. Chen, and A. Sumalee, Wardrop’s user equilibrium assignment under stochastic environment, Transport. Res. B- Meth. 45 (2011) , 534-552.
[4] X. Chen, M. K. Ng, and C. Zhang Non-Lipschitz lp-regularization and box constrained model for image restoration, IEEE Trans. Image Process. 21 (2012), 4709-4721.
[5] L. P. Jing, C. Zhang, and M. K. Ng, SNMFCA: Supervised NMF-based image classification and annotation, IEEE Trans. Image Process. 21 (2012), 4508-4521.
[6] C. Zhang, L. P. Jing, and N. Xiu, A new active set method for nonnegative matrix factorization, SIAM J. Sci. Comput. 36 (2014), A2633-A2653.
[7] R. Wang, N. Xiu, and C. Zhang, Greedy projected gradient-Newton method for large scale sparse logistic regression, IEEE Trans. Neural Learn Syst. 31 (2020), 527-538.
[8] M. Li and C. Zhang, Two-stage stochastic variational inequality arising from stochastic programming, J. Optim. Theory Appl. 186 (2020), 1-20.
[9] C. Zhang and X. Chen, A smoothing active set method for linearly constrained
non-Lipschitz nonconvex optimization, SIAM J. Optim. 30 (2020), 1-30.
[10] M. Li, C. Zhang, M. Ding, and R. Lv, A two-stage stochastic variational inequality
model for storage and dynamic distribution of medical supplies in epidemic
management, Appl. Math. Model. 102 (2022), 35-61.
[11] R. Wang, C. Zhang, L. Wang, and Y. Shao, A stochastic Nesterov’s smoothing
accelerated method for general nonsmooth constrained stochastic composite
convex optimization, J. Sci. Comput., 93 (2022), pp. 1-35.
[12] C. Zhang, X. Chen, and S. Ma, A Riemannian smoothing steepest descent method for non-
Lipschitz optimization on embedded submanifolds of Rn, to appear in Math. Oper. Res.
(2023)